Mastering Policy Learning with Causal Inference Techniques Causal Inference in Policy Learning: A Practical Guide Enhance Policy Learning with Causal Inference Insights Policy Learning Meets Causal Inference: Key Strategies Unlocking Policy Learning Through Causal Inference Methods

In the rapidly evolving landscape of data science and machine learning, policy learning has emerged as a critical tool for decision-making in various domains, from healthcare to finance. However, traditional policy learning methods often struggle with identifying causal relationships, leading to suboptimal outcomes. By integrating causal inference techniques, practitioners can unlock deeper insights and improve the effectiveness of their models. This post explores the synergy between policy learning and causal inference, offering practical strategies and actionable insights for both informational and commercial audiences. (Policy Learning, Causal Inference, Data Science)
Why Combine Policy Learning with Causal Inference?

Policy learning involves training models to make decisions based on observed data. However, correlational insights often fall short in understanding why certain outcomes occur. Causal inference bridges this gap by identifying cause-and-effect relationships, enabling more robust and reliable policies. For instance, in healthcare, understanding whether a treatment directly improves patient outcomes is crucial for policy design. (Causal Inference in Policy Learning, Decision-Making)
Key Strategies for Integrating Causal Inference in Policy Learning
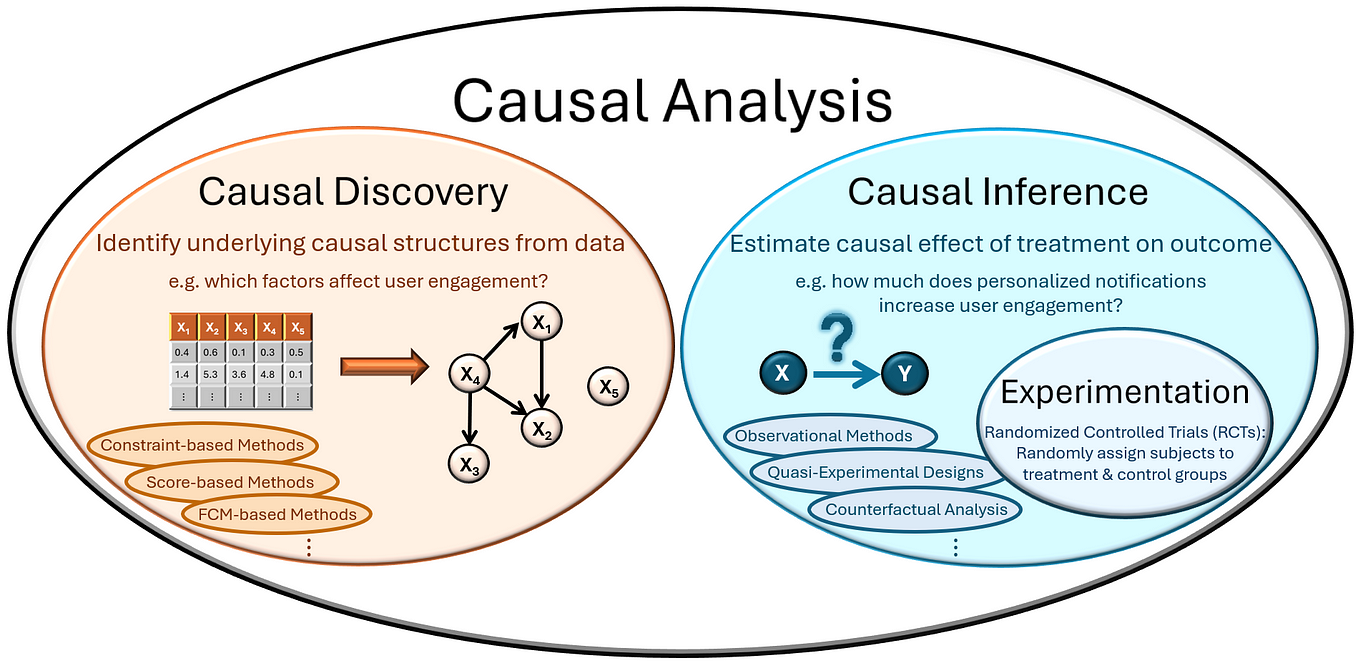
1. Leverage Propensity Score Matching
Propensity score matching helps balance treatment and control groups, reducing bias in observational studies. This technique is particularly useful in policy evaluation, where randomized controlled trials (RCTs) are impractical. By matching units based on their likelihood of receiving treatment, you can estimate causal effects more accurately. (Propensity Score Matching, Policy Evaluation)
2. Use Instrumental Variables for Confounding Adjustment
Instrumental variables (IVs) are powerful tools for addressing unobserved confounders. An IV is a variable that affects the treatment but not the outcome directly. Incorporating IVs into policy learning models ensures that causal relationships are not obscured by hidden biases. (Instrumental Variables, Confounding Adjustment)
3. Apply Structural Causal Models (SCMs)
SCMs provide a framework for representing causal relationships explicitly. By defining causal graphs, practitioners can identify and control for confounding factors, improving the validity of policy recommendations. SCMs are particularly valuable in complex systems where multiple variables interact. (Structural Causal Models, Causal Graphs)
Technique | Application | Benefit |
---|---|---|
Propensity Score Matching | Policy Evaluation | Reduces Selection Bias |
Instrumental Variables | Confounding Adjustment | Addresses Unobserved Bias |
Structural Causal Models | Complex Systems | Explicit Causal Representation |
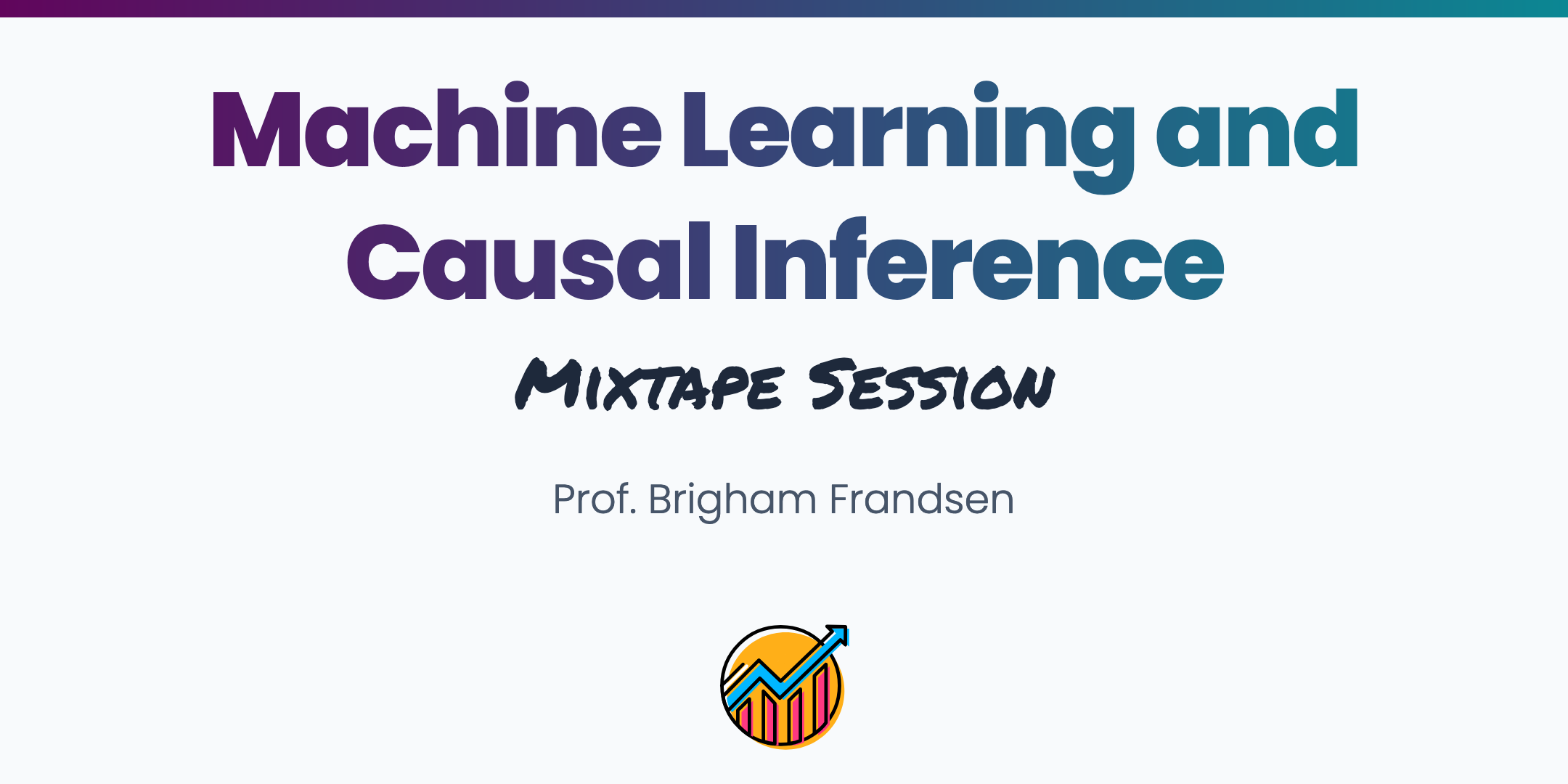
📌 Note: While causal inference enhances policy learning, it requires careful assumptions and validation. Always test the robustness of your models before deployment. (Model Validation, Robustness)
Checklist for Mastering Policy Learning with Causal Inference
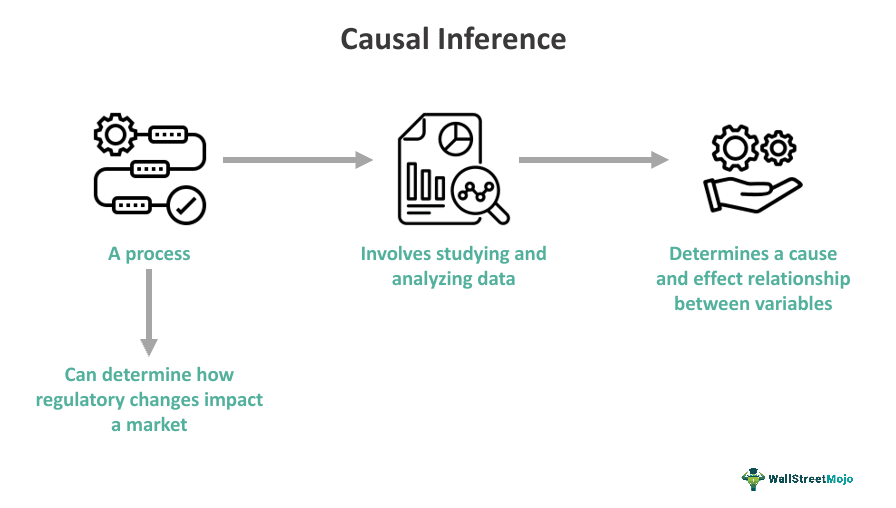
- Identify potential confounders in your dataset.
- Choose the appropriate causal inference technique based on your problem.
- Validate causal assumptions using sensitivity analyses.
- Incorporate domain knowledge to strengthen causal interpretations.
- Monitor policy outcomes and iterate for continuous improvement. (Data Analysis, Policy Outcomes)
By combining policy learning with causal inference techniques, organizations can make more informed decisions and design policies that drive meaningful impact. Whether you're optimizing business strategies or improving public services, this approach empowers you to move beyond correlations and uncover true causal relationships. Start integrating these strategies today to elevate your policy learning initiatives. (Business Strategies, Public Services)
What is causal inference in policy learning?
+
Causal inference in policy learning involves identifying cause-and-effect relationships between actions (policies) and outcomes, ensuring more accurate and reliable decision-making. (Causal Inference, Decision-Making)
Why is causal inference important for policy learning?
+
Causal inference helps distinguish between correlation and causation, enabling policymakers to design interventions that directly address root causes rather than symptoms. (Correlation vs Causation, Policy Design)
What are common techniques for causal inference in policy learning?
+
Common techniques include propensity score matching, instrumental variables, and structural causal models, each addressing specific challenges in causal analysis. (Causal Analysis, Techniques)